Models, super models and computation
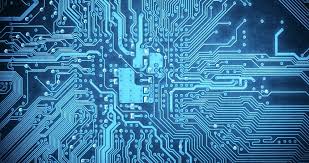
One of the first things I noticed, when I started reading about Bio-Inspired AI and neuroscience, is the notion that we cannot say that we "really" understand something unless we find a suitable "traditional" mathematical model that approximates it in a way or another.
We are so used to mathematics in physics and engineering, that without a model, no explanation is possible in those fields. We constantly look for the underlining formula, and if we do not find one, we conclude that we do not understand much, even if we can simulate it with a computer program and simple basic rules. Of course this approach has served us well for most if not all the science and technology we have developed so far.
On the one hand there are physical phenomena that we can explain within a mathematical framework, while on the other hand there are really complex phenomena (biological for example) that we have a hard time modeling with traditional mathematics, hence we consider them as being complex. I have been always intrigued by this contradiction, which has become more apparent in the last decades, with the introduction of computers and the study of biology using "engineering" approaches.
Stephen Wolfram's views about this contradiction are interesting. He does a good job explaining his views about the universe being computational and the difference between reducible (with mathematical shortcuts) and irreducible computation (the only possibility is to run the computation).
Now the question is, how do we automate the search for reducible computation? Is there a way to do it?
The second question is, how do we give the status of "acceptable" explanation to simulations of physical phenomena based on simple rules, even if we do not have a set of mathematical expressions that model it; which, by the way, is always the case in Bio-Inspired AI when we study systems with different levels of "emergence"?